A bit about me
I am an Innovation Policy Program Associate at Open Philanthropy, where I work to identify and support efforts to safely accelerate scientific progress and innovation. I am motivated by the broader goals of improving scientific research, funding, institutions, and incentive structures through creativity and experimentation, and I am always excited to connect with others who share those goals (feel free to reach out at jordan.dworkin@openphilanthropy.org!).
Before joining Open Philanthropy, I was the Metascience Program Lead at the Federation of American Scientists, and an Assistant Professor of Clinical Biostatistics at Columbia University and the New York State Psychiatric Institute. My past work has spanned US science policy, synthesis and use of research evidence, neuroimaging statistics, science of science, and mental health.
Some of my favorite recent collaborations hosting a working group of metascience policy leaders with the Institute for Progress, studying how forecasting can be used in scientific grant review with Alice Wu, developing statistical methods for multiple sclerosis research with Taki Shinohara, investigating inequities in scientific citation practices with Dani Bassett and Perry Zurn, and delving into job automation and skill networks with the folks at The Pudding. You can find more details about my work on the writing, research, and projects pages.
Side Projects
Lesion quantification toolkit
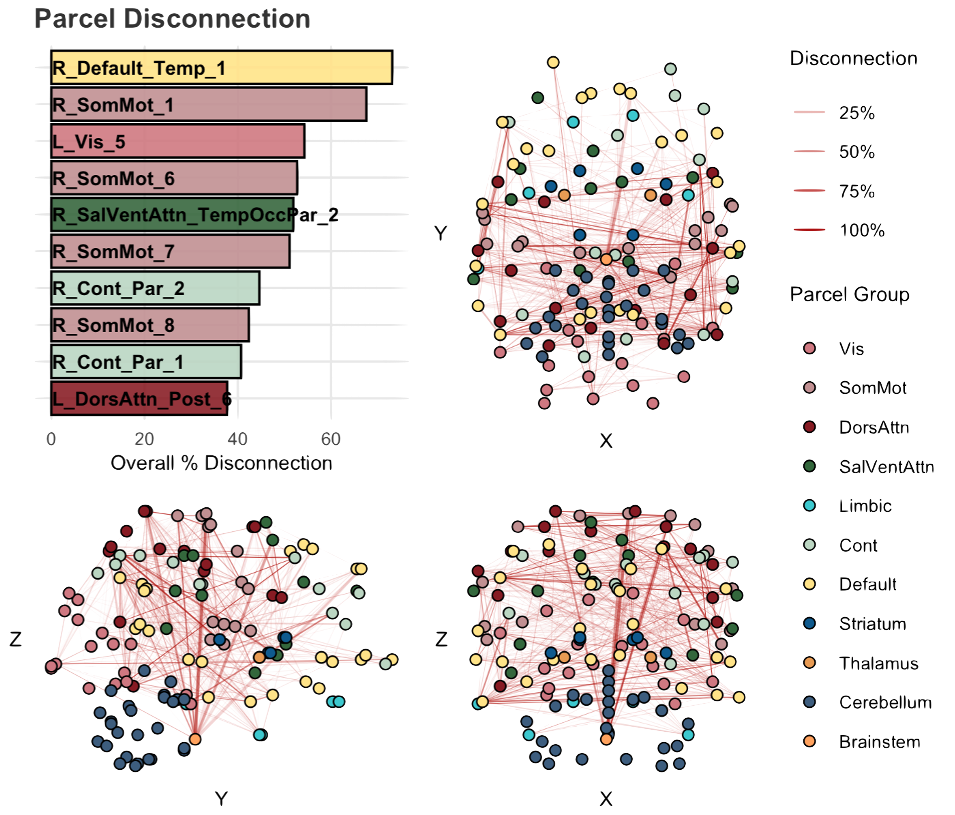
The lesion quantification toolkit (LQT) is a publicly available software package for quantifying the probabilistic impacts of focal brain lesions on structural connectivity.
Read moreSelected Publications
Citation inequity and gendered citation practices in contemporary physics
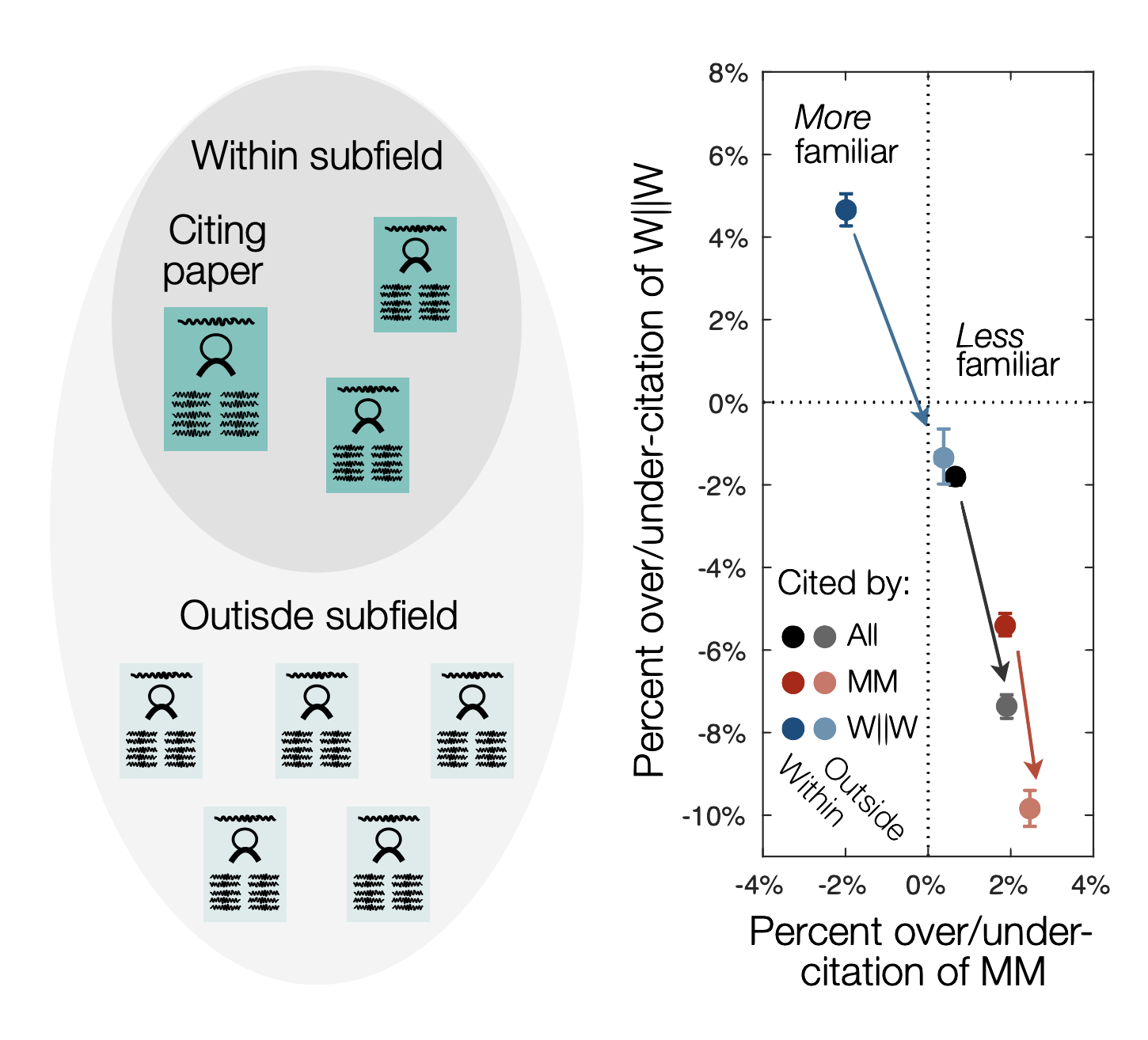
{Nature Physics, 2022}
Read moreGeneral writing
Open scientific grant proposals to advance innovation, collaboration, and evidence-based policy
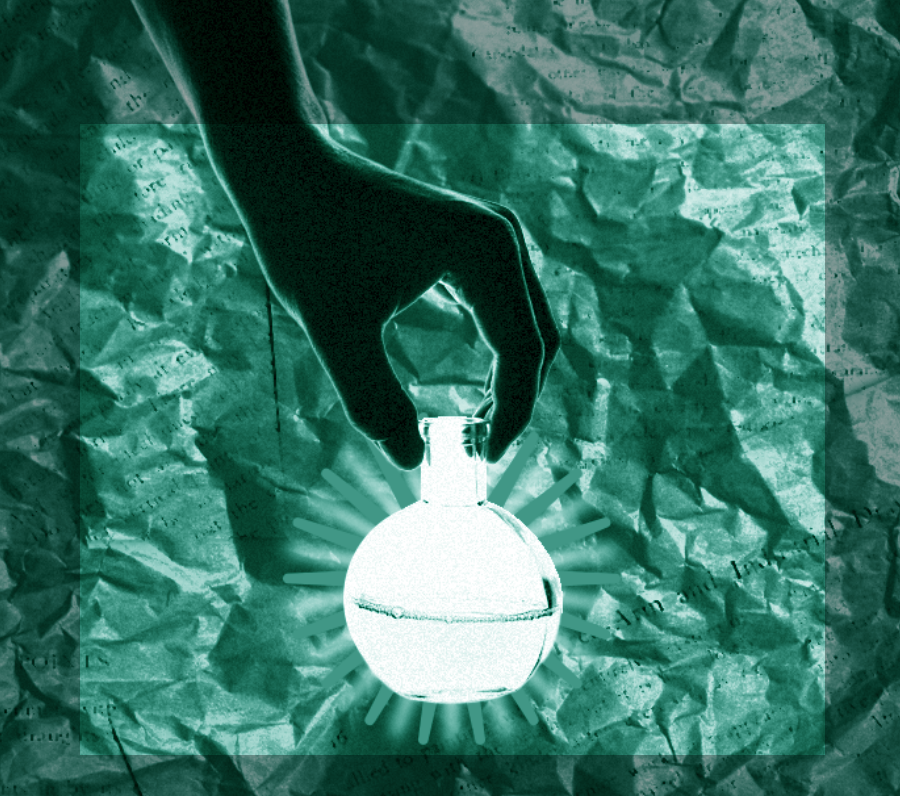
FAS Policy Memo, 2024
Read moreFeatured categories
Neuroimaging methods (6) Meta-science (4) Applied neuroimaging (3)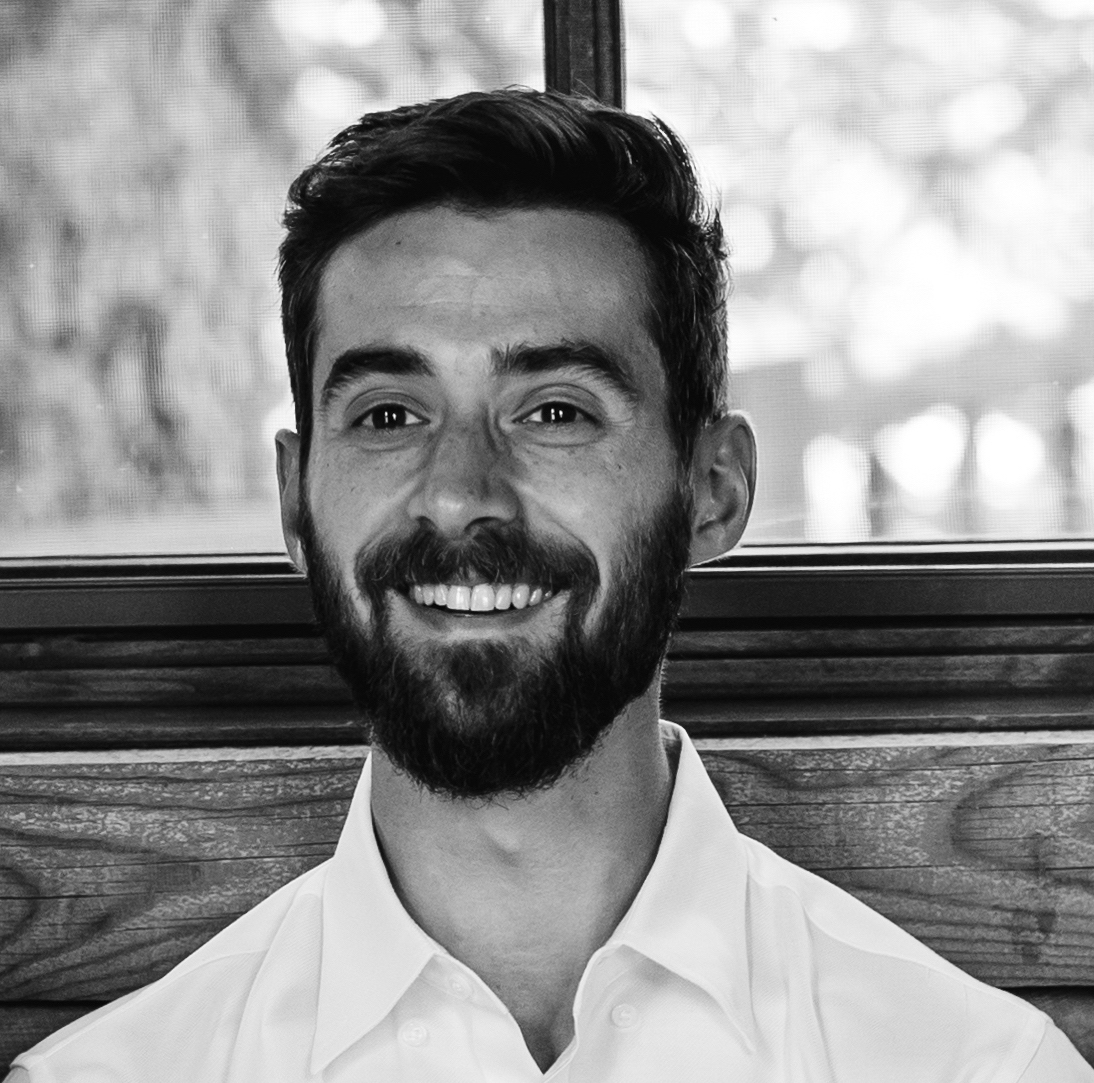